The Canary is Losing its Voice
Artificial General Intelligence, P(pretty bad), & the looming Most-Most Threshold.
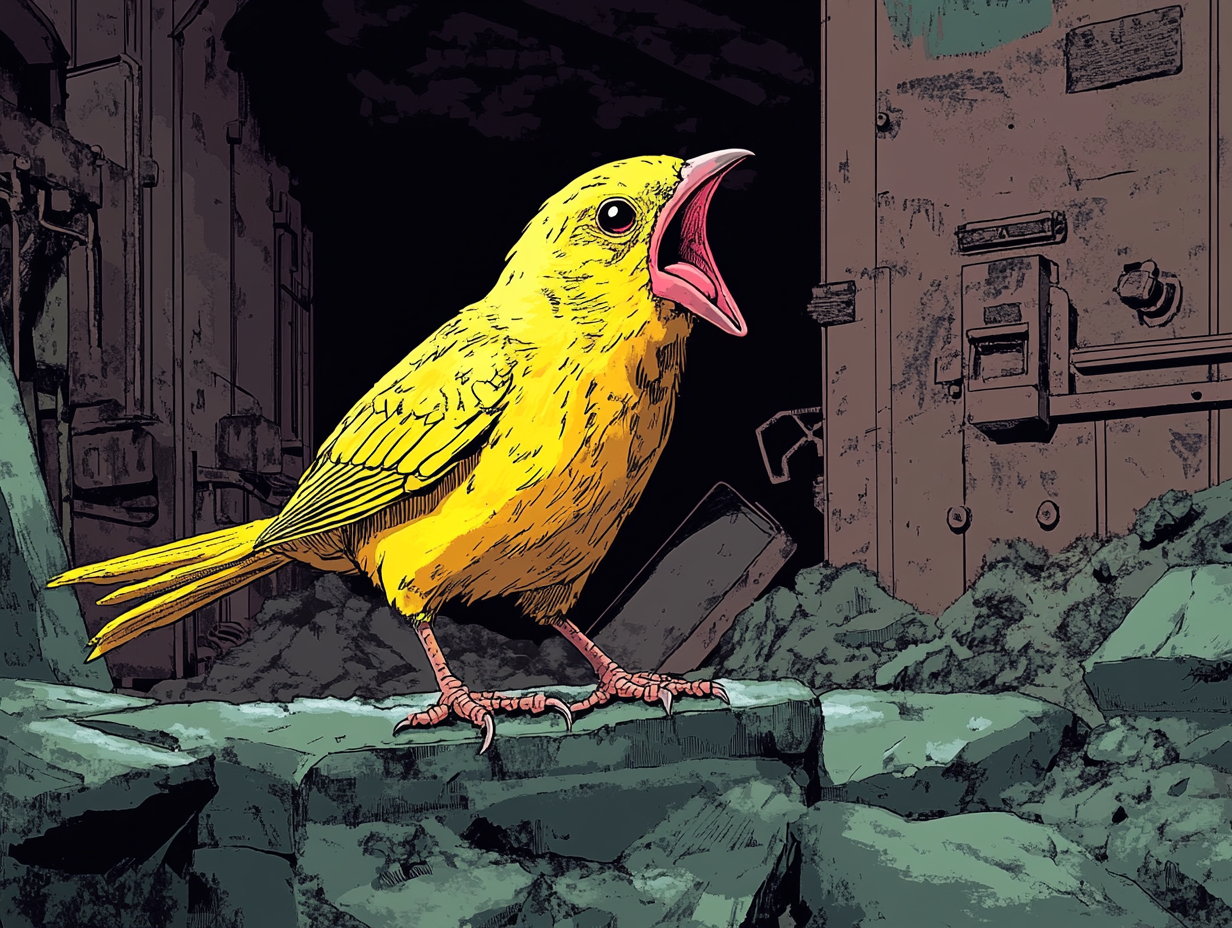
My friends know I’m a bit scared by the current trajectory of AI. Yet when asked to explain why, I often fail to do so articulately. On most attempts, my predictions and fears come out fairly jumbled.
In one of my favorite essays, Paul Graham points out that thinking without writing is incomplete thinking. So I figured, if I’m ever going to form a cogent point of view, I ought to try to put these swirling thoughts into words.
The Great Divide
Stop me if you’ve heard this one before, but the major AI labs have announced another breakthrough. Simply by adding reasoning capabilities (read: giving the system some extra time to think) the AI models have blown past industry benchmarks.
And these are the capabilities of the models they’re publicly sharing. We don’t know what still lies behind closed doors.
At this point, it’s clear we’re careening towards, well, something… Although what that “something” is is up for debate. The loudest endgame predictions tend to fall into two camps: doom (catastrophe, calamity, etc.) or a techno-utopia.
Outside the walls of the AI community, the optimists seem to get the most airtime. Think of Big Tech CEOs vying for increasingly large investments and wrestling each other for precious chips. Or Vice Presidents denouncing AI safety as an inhibitor to progress and beckoning a seemingly oxymoronic future with “pro-worker AI.”
These folks see AI as a race to the top and predict a near-term future where models are writing all our computer code, supercharging productivity, and curing disease.
On the flip side, the doomers warn that, if this pace of progress carries on much longer, we will awaken a monster. Many fear we may spawn a “fast takeoff” scenario where AI systems learn how to recursively improve themselves before rapidly reaching a point of superintelligence. In their view, humanity cannot expect to steer, much less control, an intelligence so alien and so far beyond that of our own species.
An AI apocalypse might sound like sci-fi, but you’d be surprised how seriously the community considers these scenarios. They are taken so seriously that a term was coined to represent the probability one places on catastrophe. That term is P(doom)—or the probability of doom. And, for context, in a 2023 survey of AI engineers, the average P(doom) mark was 40%… but let’s try to set that extremely unnerving point aside for a moment.
Even many on the more optimistic side of the aisle hold personal P(doom) values of 10% or higher—which, in a low-stakes game of cards, I’d count as slim. But that 10% carries a lot more weight when it’s wagering against an apocalypse.
The threats of existential doom (however plausible) are not what keep me up at night, though. Instead, what scares me is a midpoint reality that seems to be presumed by both the utopian and doom states.
I think we're now getting into the territory where models are capable enough that acting without a clear plan is irresponsible.
– Marius Hobbhahn, What's the Short Timeline Plan?
Missing the Forest for the Trees
To me, it seems as if the underlying presumption in the discourse is that we’ll first pass unscathed through a period of living with really really strong AI. And, well, it’s hard to imagine that happening—the unscathed part, that is. This is made worse because we don’t have much time to make a game plan. That really really strong AI, let’s call it AGI (artificial general intelligence), is knocking at the door.
You’d think (or hope) that a plan for a smooth transition into a new technological era was a prominent point of public debate. But, unfortunately, it feels as if nobody in power is making a plan for how to live harmoniously with something so disruptive.
At this point, the leading labs are predicting that we’ll reach AGI within the next 5 years, yet I feel that the conversation is missing the forest for the trees. If the labs are right, this would be a technology that redefines what it means to be a worker in the modern economy, let alone what it means to be a human, and there seems to be no substantive policy discussion on how to ensure a soft landing.
I can’t tell you how many founder interviews I’ve heard where, when asked about AI’s near-term future, the respondent yada yada’s social upheaval on his or her way to discussing something like AI curing disease or aligning superintelligence to human values or beating China in the race—quickly glossing over a technological transition that could be unlike anything we’ve ever seen before. It's as if they insist on saying the loud part quietly.
Maybe you (or they) can, but I simply cannot imagine a world where AI is that cognitively capable and it doesn’t spawn enormous turbulence. So as I see it, either the experts are wrong about how close we are to achieving AGI... OR we'd better start prepping.
To be clear, I do want people focusing on things like how to align future superintelligences. I do want people working to ensure this tech is not first developed by autocrats. I do want people building systems that could cure cancer. But it’d be reassuring if someone, anyone, would make a plan for what to do as AGI is rounding the final turn.
(I was heartened to see that Ezra Klein and Kevin Roose put out pieces on AGI recently. Still, it feels like, despite the warnings, there isn't adequate discussion on how to prepare for this future.)
The Most-Most Threshold
When faced with evidence that AI models are becoming increasingly intelligent, skeptics love to point out edge cases where the current models still fail miserably. So to clarify, when I say AGI is knocking at the door, I am not saying we’re on the cusp of a world where AI is better than all humans at all things. Instead, though, it seems we’re on the edge of a reality where AI is better than most humans at most cognitive labor. And, for that, I’m certain we’re not ready.
Sure, people have been saying this for years. When a computer beat Kasparov, the then chess champion of the world, futurists started shouting that the end was nigh. So by the time IBM’s Watson beat Ken Jennings at Jeopardy!, I guess the public was tired of hearing them cry wolf on human obsolescence.
The difference now, though, is twofold:
- Today’s models are exponentially more capable in an increasingly wide domain than the aforementioned.
- We have cold hard evidence that AGI is imminent. (I mean, for god’s sake, scores are rapidly rising on something called Humanity’s Last Exam.)
The moment at which we build systems that meet this criteria is what I think of as crossing The Most-Most Threshold. Get it?
Quick sidebar. I’d entertain the argument that, from a purely technological perspective, we’ve already crossed this threshold, but that the reason we don't feel it so acutely is that the tech hasn't yet been broadly adopted or deployed.
Unrelenting Interns
Crossing The Most-Most Threshold may not lead to catastrophe overnight. Much like AI progress started in the 50’s with a trickle, the impacts may be relatively small and disparate at first—a few industries disrupted here and there. With time, though, they will compound and proliferate.
Just, for a second, think about what it would mean if we invent something that is better than humans at nearly everything humans can do behind a computer. Think about what it would mean for your life personally, for the labor market, and for society in general. On some level, it’s a thought experiment so bizarre and unsettling that thinking clearly about it feels impossibly hard.
So let’s make it a bit easier to chew on. Let’s put it in terms that are more familiar, assuming you work in the so-called Knowledge Economy. (I kind of hate that term for its implication that other jobs don’t require knowledge, but anyways…)
Imagine your firm hires a new class of interns—except these interns are weird. Without any on-the-job training, they seem to know everything about the industry. Your company has a reputation for overworking and underpaying interns, but this group seems to welcome the workload. You’re not sure how it’s legal, but rumors are flying that they’re working for pennies on the dollar. And, come to think of it, you’ve never seen them go home. They’re there when you arrive. There when you leave. Always working.
The IT department typically doesn’t hire summer interns, but when you go to get help unlocking your computer, there they are. Dozens of interns coding away. Breathlessly. Never looking up from their screens.
One intern even offered to reply to all your emails. You’d normally never give someone access to your inbox, but you’ve seen how competent they are, so you figure, why not? Their replies start flowing and your head begins to spin. If you didn't know any better, you’d say you wrote the emails yourself. They’re indistinguishable from how you talk.
Then one day you swing by marketing. You’re startled to see that, instead of your usual colleagues at their desks, it's just interns designing and typing away with their unrelenting fervor.
And you can’t prove it, but you swear they’re multiplying.
This might sound like a passage of R.L. Stine, but we’re starting to see this scenario come to life.
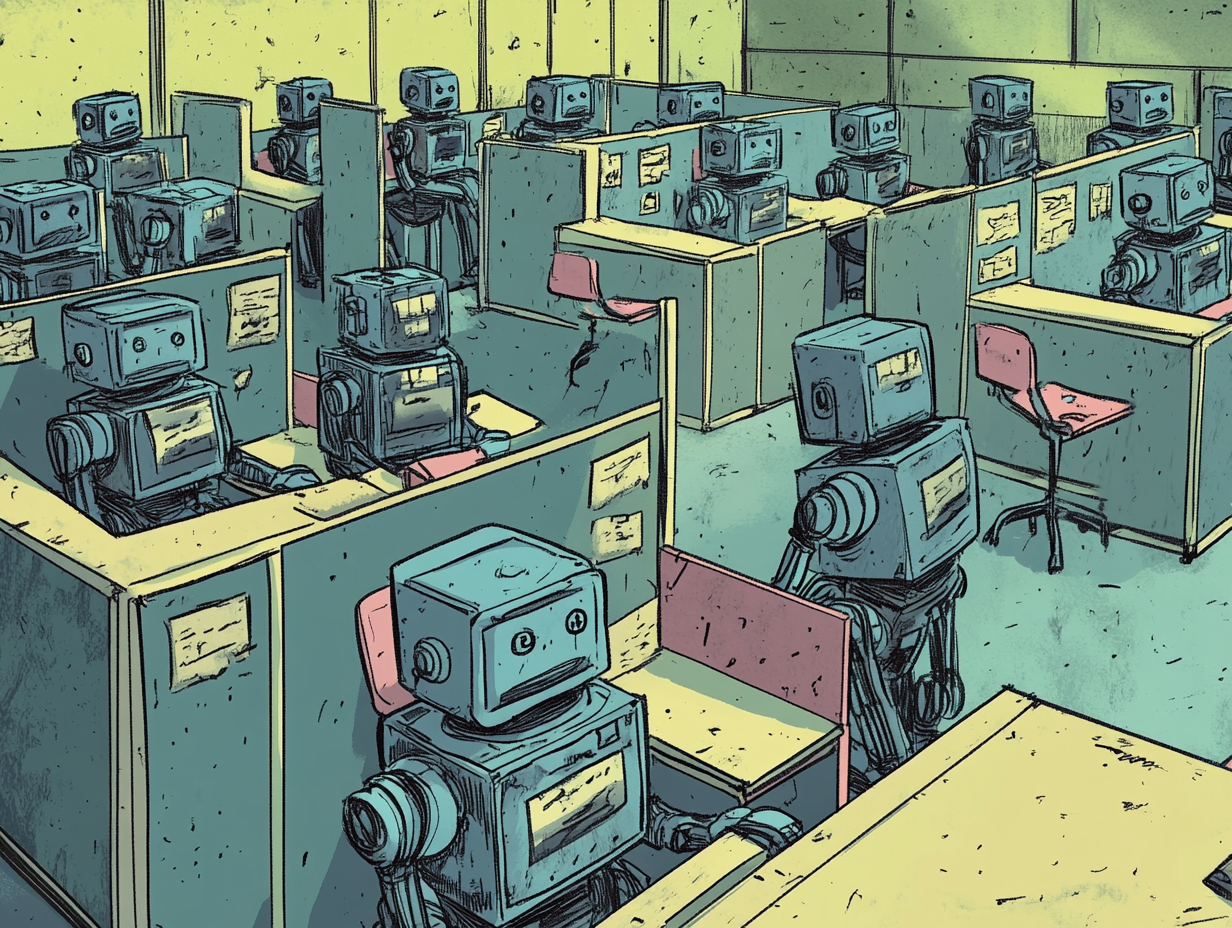
The Ocean is Receding
There have been many recent cases where CEOs have cited AI as the reason for major layoffs. For example, Workday, an HR software firm, fired 1,750 employees in the name of AI.
However, it'd be disingenuous to say that these sorts of layoffs are entirely due to AI-driven worker obsolescence. It seems that at least some of these recent waves of firings were to make room for new technical employees that will empower the firm to usher in AI solutions—an example of AI actually creating new roles.
But, again, the capability of these systems is rising so rapidly, that some disruption feels inevitable. Once they are built, models can be distributed across use cases at the speed of software, and I strongly suspect this speed will outpace the market's ability to identify new, AI-enabled roles—at least in the short term.
Speaking of, let's look at some of these capabilities...
Software Development
OpenAI’s o3 model, their premier reasoning model, now scores in the 99.8th percentile of competitive coders—essentially making it the 175th best competition coder in the world. And their CEO, Sam Altman, predicts the model may claim to the top spot in the rankings by year’s end
Of course, competition coding ≠ real-world software development, but these skills translate quite well. In fact, Mark Zuckerberg predicts that AI should be able to act effectively as a mid-level engineer in the coming months. Mid-level. Meta employs thousands of engineers.
For some context on just how fast models have improved on this front, just 3 years ago, Google DeepMind’s AlphaCode reached the 56th percentile.
Patient Diagnostics
OpenAI’s GPT-4 (released Mar 2023, and now somewhat outdated!) outperformed physicians by a wide margin in a test of patient diagnostics.
Even wilder, GPT-4 alone was more accurate than physicians who had access to GPT-4 assistance. A bit of a head-scratcher at first glance, for sure. The hypothesis here is that human physicians were unwilling to set aside initial hunches and failed to consider a wide enough range of diagnoses—whereas the models, unhindered by tunnel vision, considered a wider range of possibilities.
Psychotherapy
And, in perhaps what you might consider one of the most human domains, a recent study found that people preferred AI therapists to professional humans when the patients were asked to assess written outputs across a range of therapeutic factors.
Let that one sink in and then call your therapist.
How Soon is Now?
Until recently, most experts in the field predicted that AGI was a feat we’d achieve somewhere around 2050. The blistering pace of the past 4 years has Silicon Valley singing a different tune, though. Now, experts see this as a threshold we’ll cross within the next 5 years—with many saying it could be as early as 2026.
Take this graphic from Anthropic, one of the premier (and often most pragmatic) AI labs, for example.
They are predicting that by the end of this year we’ll have direct access to AI collaborators on par with experts in various fields. By 2027, they predict Claude (their flagship series of models) will be independently achieving breakthroughs “that would have taken teams years to achieve.” Incredible, yes, but like, what could that possibly mean for the rest of us?
Let’s take the field of molecular biology as an example. If we soon invent AI agents that are on par with Nobel prize winners, what does that mean? To me, at best, the agents would make some molecular biologists redundant and, more likely, they’d make a lot of molecular biologists obsolete. Now copy-paste that onto accountants, lawyers, copywriters, graphic designers, and so on.
In spite of, what seems to me, damning evidence of an impending economic earthquake, it’s rarely talked about in those terms by industry leaders.
In a recent interview, the CEO of Scale AI—a firm that, in simplest terms, helps train the latest AI models—said that he sees a world where “everyone becomes managers” with each employee tasked with overseeing 10 AI agents. And, who I am to say he’s wrong (or say any of this, really), but taking Occam’s Razor to this one, which scenario feels more likely:
- Firms maintain current levels of employment and each employee is handed 10 AI agents. Production improves. Profits climb.
OR
- Firms stop hiring entry level employees, start firing low performers, and each remaining department head is handed 40 AI agents. Production flourishes. Profits explode.
I’m far from an economist, so I won’t try to play one online, but one of those scenarios feels much more likely to me.
Human obsolescence feels especially inevitable as the cost gap between workers and AI continues to grow. Since GPT-3.5 burst onto the scene, the cost to run state-of-the-art models has fallen precipitously. These cost efficiencies have become so regular that a common point of advice for those building with AI is to ignore how much your system costs today—because 3-6 months from now it will cost a fraction of that.
P(pretty bad)?
It doesn’t quite have the same ring to it, but instead of P(doom), what if we talked about P(pretty bad)—that is, the probability that AGI leads to some non-catastrophic, pretty bad social and economic outcomes. Like, instead of a superintelligence unleashing a swarm of nanobots, let’s consider the possibility of mass layoffs.
This next part is by no means a statistically sound extrapolation, so put down your pitchfork.
If AI researchers assign a probability of FORTY percent to a doomsday scenario, you have to imagine their respective P(pretty bad) would be, what? 50%? 60%?
Of course, it could be that these experts see doom as a quite singular scenario where as soon as things go a little bad, they’ll go really bad really fast. And that actually the remaining room of their mental probability is spread out between decent to utopian outcomes. Even so, let’s say the consensus is a flip of a coin for a pretty bad outcome, that seems likely enough to warrant a discussion.
I’m fairly certain that leaders in AI see this economic upheaval on the horizon as or more clearly than I do. Though what incentive do they have to raise the alarm? If they instill the fear of AGI into too many policymakers they risk being shackled by regulation. And regulation in one country just opens the door for firms in others to charge ahead. So what good does that do?
In D.C., the main concern seems to be ensuring China doesn’t beat us—which, again, I’m all for. Though, I also think it’s the elected officials’ duty to identify the potential harm in anyone achieving AGI and to prepare accordingly.
Plus, if the labs are confident AGI will soon help us cure cancer or something of the sorts, a bit of collateral damage to the job market might feel like a small price to pay.
For what it's worth, I'm on the side of the AI firms on this one. The potential upsides of the technology are so large that I do not think we should try to slow down progress. We just need other folks to put an equal amount of energy into ensuring a smooth transition.
It is not the founders' responsibility to come up with a plan. That's what we have policymakers for. After all, I don’t think anyone tasked Gutenberg with ensuring scribes found new jobs after he unveiled his printing press.
So onward we march.
So… what?
It’s at this point one would expect me to share some ideas on how we can avoid this upheaval, but I honestly don't have too many.
Universal Basic Income feels like a good place to start. We’ve heard people refer to building towards a pro-worker AI, but it doesn’t seem like anyone has described, in pragmatic terms, what that would actually mean. Guaranteeing that those caught in the first few waves of obsolescence have an income to fall back on seems rational.
We’ve seen some evidence that humans, when helped by AI, outperform AI systems in isolation. But, I have also pointed out an example where models alone beat doctors who had access to AI—presumably because the doctors were not using the models to their fullest capability. To me, this suggests that investing heavily in AI education—that is teaching people how to most effectively use AI to supplement their work—may be a good game plan.
This could come to life as required coursework in all university tracks. “AI for [insert a major]” could be taught such that we ensure future workers are leveraging the human + machine dynamic to the fullest.
And for those who are currently employed? I'd hope that more firms begin training their staff on how to best use AI. But you don't have to wait until your company wakes up—start using it yourself!
Of course, I could be wrong about all this. Perhaps AGI as its currently defined is never achieved. Or perhaps we smoothly transition into this new reality. Or perhaps it takes firms a really long time to figure out how to deploy it across their businesses. All are totally within the realm of possibility.
To my earlier point, though, on some level I believe the models we currently have access to are strong enough to cause some real trouble—we just haven't seen enough businesses catch on yet.
Ethan Mollick, one of today's preeminent writers on AI, points out that even if the big labs suddenly stopped making improvements, it'd likely still take us years to figure out how to optimally use the models we currently have at our disposal. Capability doesn't appear to be slowing down any time soon, and I suspect that the intelligence curve and cost curve will accelerate the adoption curve.
I genuinely am hopeful that AI will enable us to cure disease, usher in a new era of space exploration, and reduce human suffering. But unless we figure out how to make the impending transition a smooth one, I can’t help but feel that we’re in for a world of hurt.
Big shoutout to Ezra Klein, Kevin Roose, Marius Hobbhahn, Ethan Mollick, and Dario Amodei for inspiring this post and always giving me something good to read.