How AI is Changing the Way We Shop
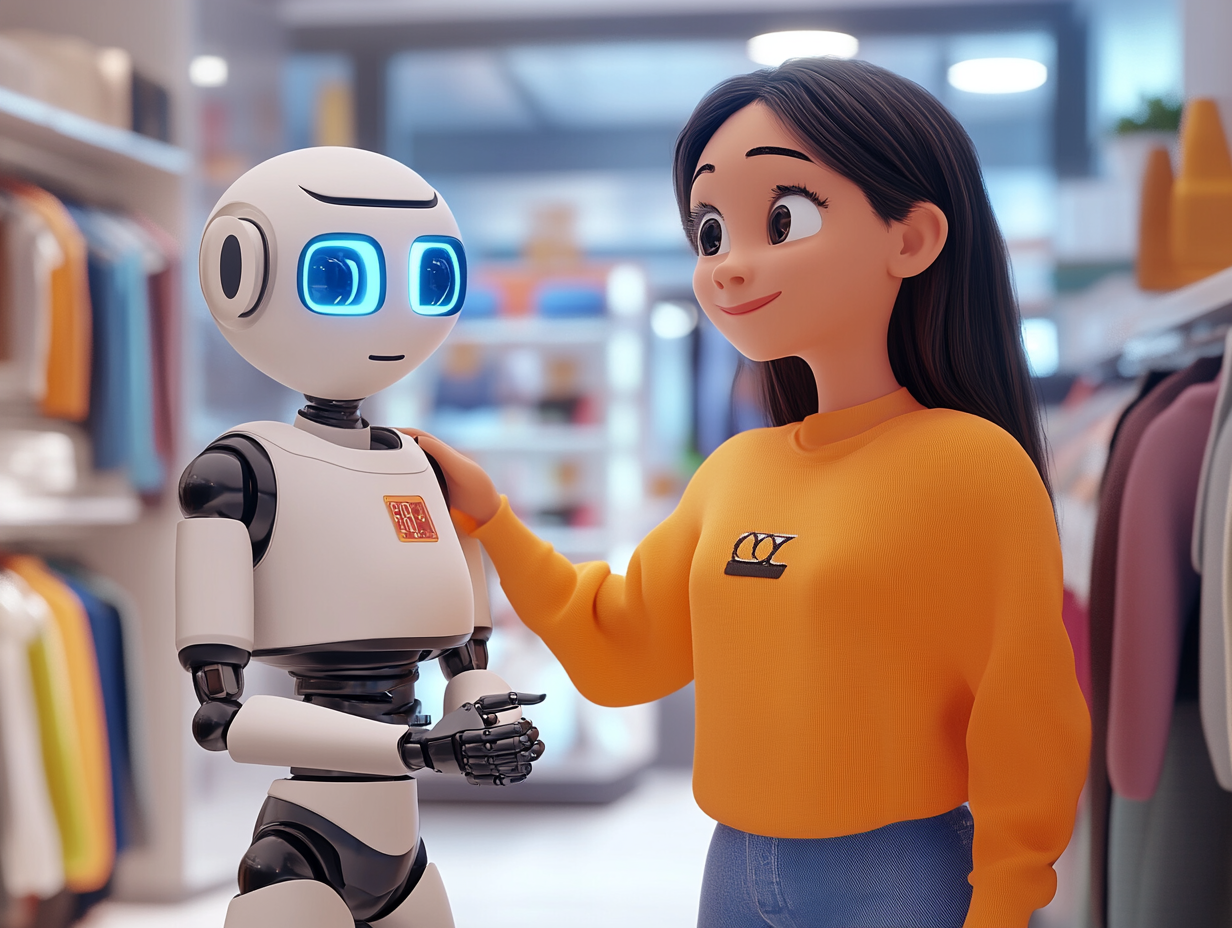
A few years ago, I would have guessed that by Feb 2025 generative AI would play a larger role in the online shopping experience. Like most applications of the technology, though, AI in the retail arena has progressed in a series of fits and starts. We’ve seen many companies (Outdone included) launch splashy AI-powered shopping tools just to find that they come up short in critical ways—stymieing adoption and leaving most customers reaching for more familiar alternatives.
That said, whether you see it or not, AI is all around us. In fact, most of the uses for AI in e-commerce actually operate behind the scenes, providing value to shoppers without them even realizing it—coming in the form of more capable search engines, faster shipping times, and predictive recommendations.
And although we don’t yet have omniscient and agentic personal shoppers at our fingertips, I’m quite confident that’s a reality that is just around the corner. (Maybe I’ll be right this time.)
The Promise (and Current Reality) of AI Shopping Assistants
As the founder of an AI-powered product recommender, there were two recent launches that made me hold my breath. These were Amazon’s Rufus shopping bot and Etsy’s Gift Mode. Both would inevitably compete directly with Outdone—and, of course, both had the manpower and the purse of a huge firm behind them.
But when the dust settled from the launches, neither saw huge user adoption. In fact, Rufus continues to receive less than favorable reviews and Etsy’s Gift Mode has been quietly shuttered less than a year after its debut.
Users of these and other AI shopping assistants (again, Outdone included) have largely reported that the AI tends to underwhelm. The bots are capable of delivering okay, even good recommendations in many cases, but fail to wow users in ways they’d expect.
Why Even the Top Dogs Struggle
So what went wrong and what does that mean for the future of AI shopping assistants? Well, I can’t claim to have all the answers. If I did, Outdone would be on a one way ticket to the moon. We’re still struggling to figure it out ourselves.
I do have some theories, though:
- Retailers are relying too heavily on Generative AI—a technology that wasn’t built to be a recommender system. Each series of new models seems to be improving on the recommendation front, but there are many frameworks that still outperform it when it comes to suggesting products to users.
- Assistants are only as good as the products they can recommend. You wouldn’t ask a dollar store clerk for the best air fryer. That’s not in their inventory. The same is true of Amazon, Etsy, or Outdone. If the perfect product for that user isn't on the site, even the best AI would come up short.
- Shoppers struggle to distill their preferences into words. GenAI is great at taking language and replying with informed, personalized, and friendly words. But when it comes to buying a new couch or sweater, it’s really hard to find the words to describe what you’re looking for in the first place—and why one couch has that je ne sais quoi and the other is hideous.
Until the LLMs become the super intelligences that many of their developers claim they’ll be, the best AI-powered shopping experiences will likely come from a combination of LLMs, computer vision, and more traditional (but powerful in their own right) recommender systems. Now it’s just a matter of who will be the first to conjure the correct recipe of modalities, products, and assistance.
But enough about shortcomings, let’s talk about some ways AI has truly changed the shopping game.
The Search Revolution: A True AI Success Story
In the not-so-distant past, if you were to search for a green couch, the only results that would appear were ones where the words “green” and “couch” were prominently featured in the product name, description, or tags. For online retailers, this required a painstaking process of manually ensuring that every item was labeled properly.
Those days are over. Now when you search “green couch,” you’ll see results like a “Modern Style Sofa” in "Olive Corduroy." This is thanks to advancements in semantic search. The best search engines now understand that “green couch” and “olive sofa” essentially mean the same thing, even if the literal words are slightly different.
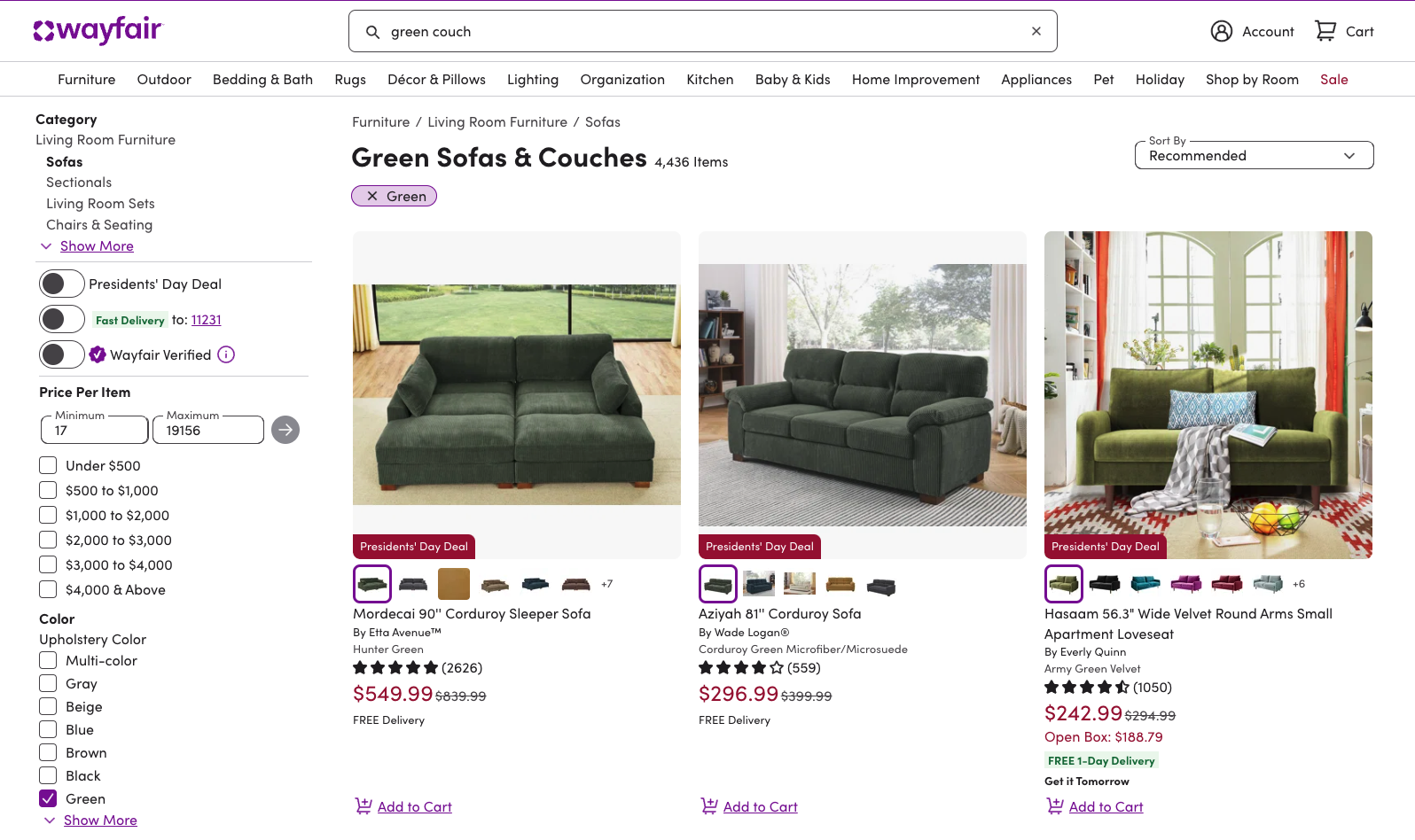
Improvements like this take the onus off the shopper to precisely describe what they’re looking for, and allow for simpler, easier shopping.
Visual Search: High-End Inspiration to Affordable Alternatives
But things go even further. In many cases, you don’t even need to use words. Thanks to improvements in computer vision and image embeddings, you can simply take a photo of a product—say a lamp in the display window of a super high-end store—and use the image to find cheaper alternatives in the same style.
The Want/Need Shopping Dichotomy
Because search has gotten smarter and more efficient, finding commodities, where quality and style is unimportant, has become insanely easy.
This afternoon I realized I needed a USB to USB C adapter for an old thumb drive I had in my desk. I could have taken the 5 extra seconds to word the search properly, but instead I just entered “thumb drive converter thing” into Amazon—and low and behold, the top result was exactly what I was looking for: a $9 piece of plastic from some no-name brand. We can thank improvements in machine learning for that one.
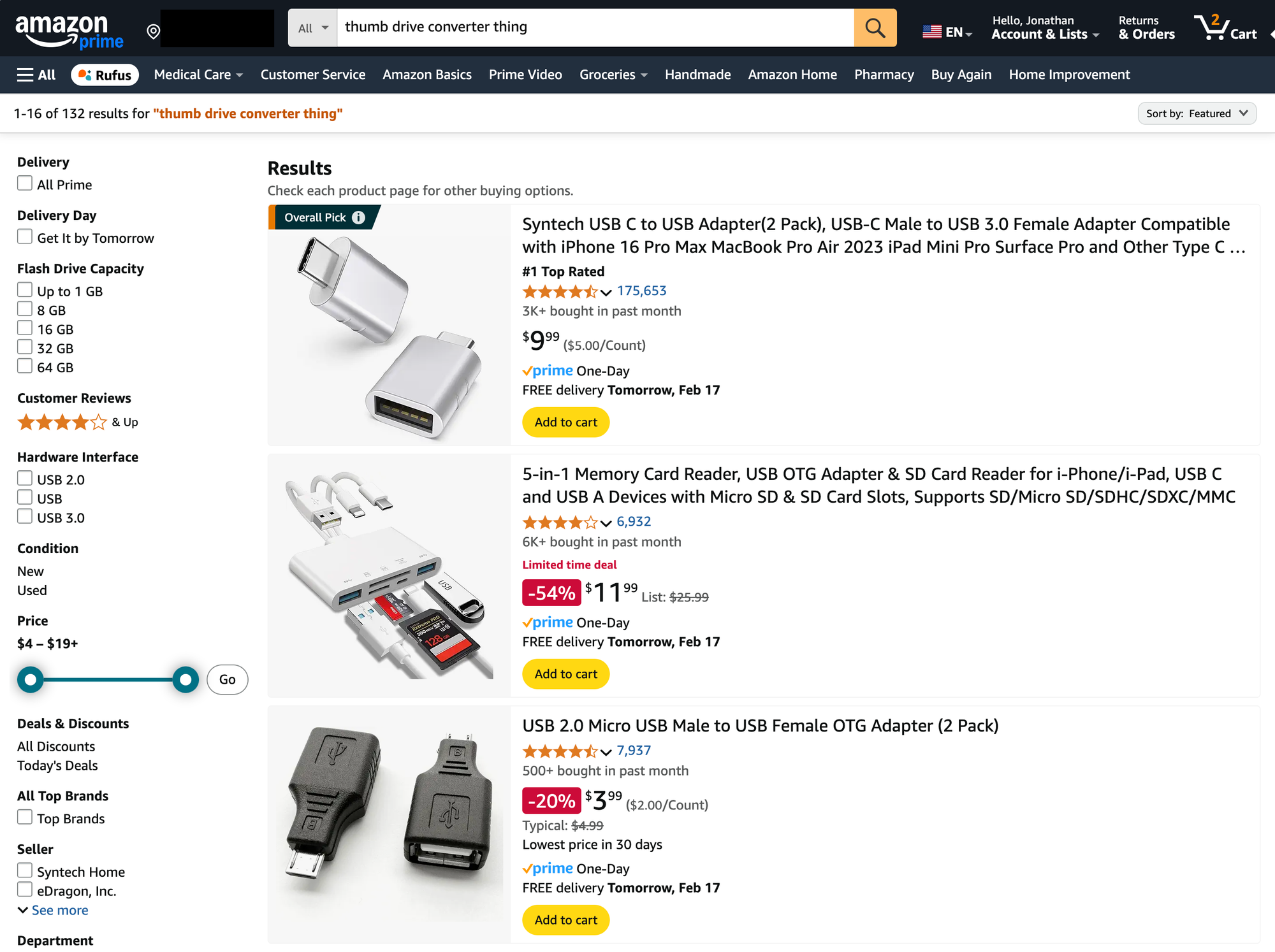
The same is not true, though, when I’m shopping for a new sweater. That’s because when shopping for something I don’t necessarily need, I typically enter the purchase journey without a clear idea of what I’ll come out the other end with. And I think for that reason, shopping for wants is much harder to solve with AI. There isn’t a correct answer, per se, that the AI can identify. And even when you find one you love, it’s really hard to put into words why it’s the perfect sweater for you at that moment.
The individuality of style when it comes to clothing, home goods, or outdoor gear is an area we’re still actively trying to crack with AI, but I predict shopping for commodities will soon become a chore of the past. I mean, it’s hardly even a chore at this point, yet I think it will get even easier.
The Future of Buying Basic Necessities
Agentic systems should be so capable in the near future such that whenever you need batteries or paper towels, you can simply shoot a message to your AI assistant and it will automatically order some to your house—at the lowest price and with your brand preferences in mind. (The price thing is something it currently struggles with. 😅)
Once this is a well-oiled machine, it’s not hard to imagine that these agents will actually predict when you need to place those orders, so you don’t even have to bother asking.
When AI Customer Service Works (and When It Doesn’t)
Okay, back to the sweater example. Say you find the perfect one after searching for days. You hit order and patiently wait for it to show up. But when you try it on for the first time, you realize it’s the wrong size. Brutal. Time to make a return.
With each passing month, more firms are relying on chatbots to handle customer service requests like these. And, admittedly, it’s becoming harder and harder to discern when you’re speaking with a human or a computer.
I’m not sure Alan Turing had online customer service in mind when building his machine, but today’s LLM-powered service agents sure do pass his Test (in most cases).
Still, the AI representatives tend to fall short when it comes to more complex or unique service requests. Need help returning a shirt or finding an order number? The AI can handle that no problem. But try explaining to a Ticketmaster chatbot why your seats that were supposed to be next to each other are actually three rows apart, and you'll quickly find yourself longing for human intervention.
The Jagged Frontier of Progress
Progress in AI is often referred to as a “jagged frontier,” meaning improvements (and stubborn shortcomings) often occur in unexpected ways. One of the most head-scratching examples of this is how LLMs can’t tell time when shown a picture of a clock. These are the same models that can look at a static web design and write code for a functional web app in like 30 seconds.
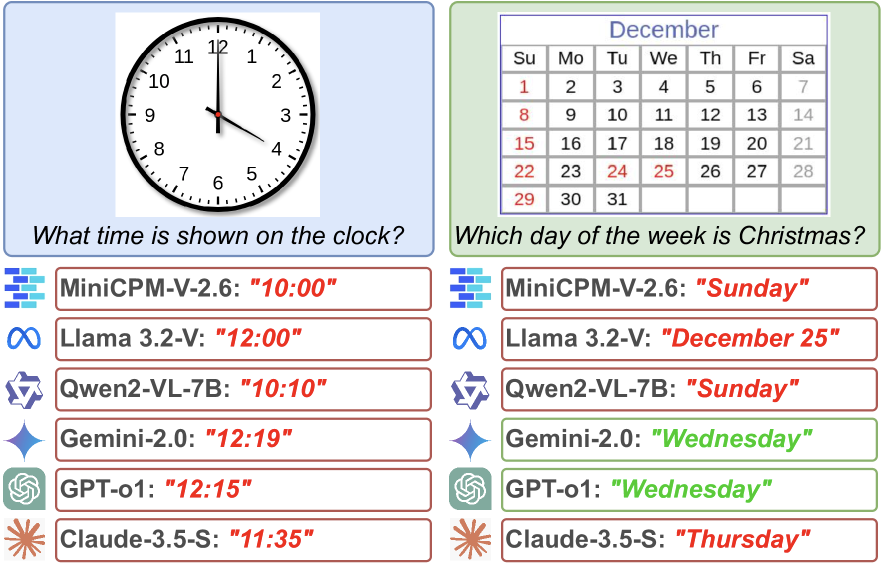
Yet despite these peculiar limitations, to me, the trajectory is clear on the customer service front. I'm confident that nearly 100% of online customer service will be handled by AI by year's end.
Using language to solve problems is an LLM's bread & butter. Add on the recent advancements in AI reasoning (which would help solve trickier problems like the concert ticket mishap) plus the sheer amount of training data available in the customer service domain, and you’re looking at a use case ripe for AI overhaul.
What’s Next for AI Shopping
While predicting how and at what speed AI will progress has proven to be extremely difficult, I think we all can agree that companies are going to try their damndest to weave AI into more retail experiences. The prize for the taking is simply too large to not throw everything at the wall to see what sticks. According to Statista, global e-commerce revenue is expected to land north of $4T in 2025.
Pair that massive number with the fact that AI is enabling companies to ship new features faster and more cheaply than ever, and you’re looking at an enormous ROI opportunity.
So don’t be surprised or alarmed if the next time you head to your favorite retailer, you find an AI bot asking if you need some help.